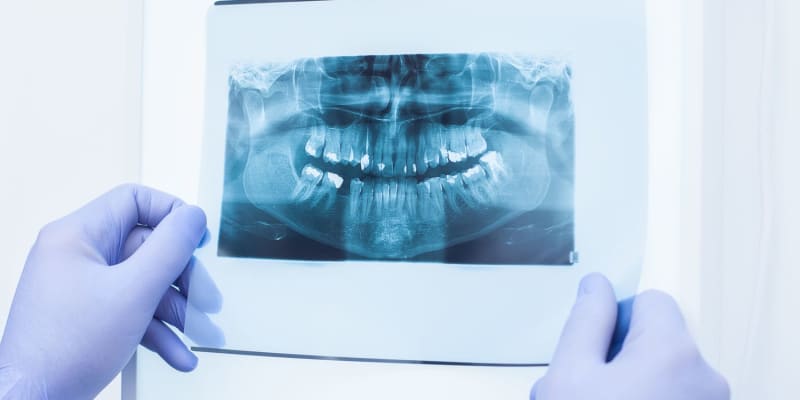
Researchers in Brazil created a machine-learning system that can determine an individual’s sex based on panoramic radiographs – wide-view dental X-ray images that capture the entire mouth. The system was 96% accurate when the resolution of the picture was good and the individual was older than 16. The accuracy was lower for younger individuals. The their findings were published in the Journal of Forensic Sciences.
When forensic experts need to identify an individual based on human remains, determining the sex of the deceased is one of the first goals. Throughout history, various methods have been used for this, many based on the analysis of bones, their structures, and relationships. Forensic methods that extract the needed information from bones are particularly important because bones are often the best-preserved part of a body.
Sometimes forensic experts have only the jaw and teeth to work with. This is particularly the case in mass accidents or other situations where analyzing human bodies is in different stages of decomposition, e.g., bodies that are charred, macerated, or skeletonized. These are situations where techniques of forensic dentistry are of utmost importance.
Like many other areas of human activity, artificial intelligence (AI) is making inroads in this area as well. AI-powered methods can assist dentists in interpreting images more effectively, reducing human errors, and allowing for faster determination. It is also possible that AI-based techniques could be used to obtain information that might not be easily detectable to the naked eye or using classical methods.
Study author Ana Claudia Martins Ciconelle and her colleagues wanted to explore whether an AI-powered tool could be developed that can accurately estimate the sex of an individual based on a panoramic radiograph. Panoramic radiographs are wide-view dental X-ray images that capture the entire mouth, including the teeth, jaws, and surrounding structures, in a single, comprehensive picture.
These researchers collected 207,946 panoramic radiographs and their corresponding reports from 15 clinical centers in São Paulo, Brazil. These panoramic radiographs were acquired using four different devices. Fifty-eight percent of them came from female patients. All patients were alive at the time the radiograph was made. Forty-three percent of them had up to four missing teeth. Five percent had more than sixteen missing teeth.
The study authors organized data from each patient into a database and trained two machine-learning algorithms to estimate the sex based on a panoramic radiograph. One algorithm was a convolutional neural network, while the other was a residual network. A convolutional neural network is a type of deep learning model that learns hierarchies of features in a set of input images. A residual network is a type of deep learning model that uses shortcuts to help pass information through layers more effectively.
Results showed that, after optimization, both types of algorithms had similar accuracy in estimating gender. The main factor affecting the accuracy was the resolution of the pictures—when it was better, accuracy was higher. The second important factor was age. For patients between 20 and 50 years of age, the accuracy of the system was over 97%. It was a bit below 95% for patients above 70 years of age.
For patients between 6 and 16 years of age, the system was 87% accurate in estimating their sex, while accuracy was only 74% for children below six. For individuals over 16 years of age, the overall accuracy was 96%.
“This study demonstrates the effectiveness of an AI-driven tool for sex determination using PR [panoramic radiographs] and emphasizes the role of image resolution, age, and sex in determining the algorithm’s performance,” the study authors concluded.
The study presents a new software tool for determining the sex of an individual based on panoramic radiographs. However, it should be noted that all the images used in the study came from people who were alive. The effectiveness might be different if images came from human remains where decomposition processes have already advanced.
The paper, “Deep learning for sex determination: Analyzing over 200,000 panoramic radiographs,” was authored by Ana Claudia Martins Ciconelle, Renan Lucio Berbel da Silva, Jun Ho Kim, Bruno Aragão Rocha, Dênis Gonçalves dos Santos, Luis Gustavo Rocha Vianna, Luma Gallacio Gomes Ferreira, Vinícius Henrique Pereira dos Santos, Jeferson Orofino Costa, and Renato Vicente.